Table of Contents
- Please share with us the history and background that led you to write this paper
- What's the new approach in decision-making that is currently lacking in the world of electronic health records?
- If something goes wrong because of the system, then who will be sued?
- Are there any practical complications for the existence of this system?
Status
Published
Video
Video
Ready to Publish
Ready to Publish
Publish Date
Mar 3, 2021
Target
Long Form
Blog Ideas
Design
Drafted
Graphics
Graphics
Type
YouTube
Video Recording
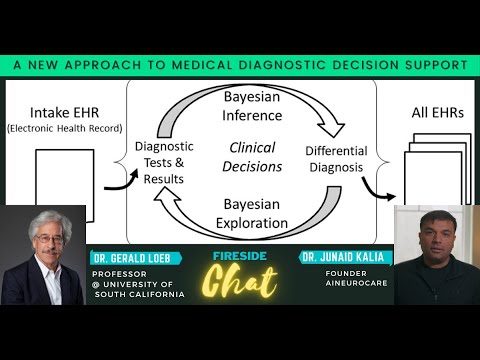
During this pandemic, when more and more clinicians are pushed to burnout, it should be our utmost priority to relieve our soldiers/fighters from this ever-growing pain of exhausting work. Technology has been the helping hand of Clinicians from day 1. But it has never achieved the level yet to support them with the iterative as well as patient-clinician-based tips and suggestions. There is a long way for knowledge-based & pattern-based AI to reach this level of support in differential diagnosis. This is what intrigued Dr. Loeb in writing his recent article titled “A new approach to medical diagnostic decision support”. So, in this video podcast, we have the pleasure of having Dr. Generd Loeb discuss deeply on this topic. FYI, he is a professor of Biomedical Engineering at the University of Southern California and also is the Director of the Medical Device Development Facility.
According to Dr. Lobe, this support system will be like a well-experienced scrub nurse, where she knows your style of operating and also knows what step you are gonna take next. So, she will hand out the instruments even before you reach out to her for them. Similarly, his vision is of a support system that will suggest Clinicians with questions and tips to ensure that he or she treats the patient properly.
The following were some of the interesting topics that were discussed in the podcast.
- Background and History that led Dr. Loeb to write this paper
- What's the new approach in decision-making that is currently lacking in the world of electronic health records?
- If something goes wrong because of the system, then who will be sued?
- Are there any practical complications for the existence of this system?
- Explaining Knowledge-based & Pattern-Based Artificial Intelligence. And how does Dr. Loeb’s new approach differ from them?
- Why Is EHR Data Unstructured and what are its consequences?
- What is the probability distribution function of diagnosis and tests and how do the matrices come to play?
- How far are we in introducing blockchain and coinification in the healthcare industry?
- The effectiveness of this System in Terms of Cost and Benefit
- Will there be a need for the Medical Society to intervene with the probabilistic results of Systems?
- The Need for New Approach by FDA over Softwares in Medicine
So, let’s go through Dr. Loeb’s vision and ideas by knowing his response to our questions.
Please share with us the history and background that led you to write this paper
I've been fascinated with the process of differential diagnosis since it was taught to me as a medical student at Johns Hopkins. It was taught to me as a very logical procedure in which one collects all the facts and arrives at a conclusion by deduction. And it's been surprising to me how little of that is actually able to be addressed using the very powerful tools that have been developed. Machine learning, artificial intelligence, lots of ideas have been out there. But it seems frustrating to me both, through my limited experience as a practicing physician, and my experience as a patient. So about 20 years ago, we got involved in how the brain receives and processes information about touch. And that got us to realize that the tactile information coming from the skin or from an artificial tactile receptor set that we developed for robotic hands wouldn't generate any useful information until you made an exploratory action. And then we realized that we needed a system to systematically decide on those exploratory actions in a way that you could incrementally explore objects and identify them based on prior experience. And after we developed that, and achieved fairly remarkable success with it in terms of identifying objects by their haptic properties, I realized that that was essentially the same process as differential diagnosis. You start with a patient with a set of symptoms, and maybe some initial findings. And then by process of incremental, deciding on what you do next in the examination, what tests you order next, you arrive at what hopefully will be the correct diagnosis. And so having realized that they were the same processes. I've been working for the last few years to think about what the practical problems are, to take that solution, which works very well for the very limited problem of haptics and adapted to the much more complex world of medical diagnosis.
What's the new approach in decision-making that is currently lacking in the world of electronic health records?
Bayesian inference, which my algorithm absolutely depends on, is the forward direction of saying, given this test result, what are the probabilities of these various differential diagnoses? What Bayesian exploration does is it says, given all these relative prior probabilities, which next test is most likely to disambiguate them. So, it allows you to preserve all the possibilities, the next test may increase or reduce those probabilities. You don't forget about all the possibilities, you just gradually refine their probabilities. If you think about it, that's what we do. When we're trying to identify an object. We take whatever prior information we have. And in our mind's eye, the possibilities emerge. And then as we make exploratory movements, and collect more data, we gradually refine the probabilities always prepared for a surprise, which then forces us back to change our minds and say that something that we discounted previously is, in fact, more likely to be the case. And basically, exploration is actually a mathematically optimal way to make that decision about what to do next. And then as a result of that, the next turn in the cycle, compute new posterior probabilities, and then decide if they're high enough to conclude this is an acceptable diagnosis, or if, in fact, further testing is required. And that further testing again, now suggests what would be the most effective test to do next to further resolve the probabilities. That really hasn't been done before.
If something goes wrong because of the system, then who will be sued?
The whole notion of a ‘requirement’ section came naturally to me because I come from the world of medical devices. And in medical devices, all class-two and class-three medical devices do impose some risk and are required to do something called design controls, in which you define all the requirements from all the stakeholders, in all the circumstances of use. Before you implement any large, complex system, you have to do that to make sure that what you produce is fit for purpose. And the stakeholders are a very broad set of folks, it has to be something that meets the patient's needs, obviously, getting to a correct diagnosis and treatment, the physician’s needs which include making their time more efficient, and protecting them from liability. It has to be technologically feasible and engineers and programmers have to know that they have the required tools. And so very quickly, it became clear that what's done in engineering to help technicians service jet engines for instance, which is a very effective use of AI, isn't gonna work in medicine. Because the physician needs a lot of subjective judgment and also needs a personal relationship with the patient. And I think that this system addresses all of those because it's generating suggestions, rather than requirements, it avoids one source of the problem. And because those suggestions are not based on expert opinion, they're based on the totality of electronic health records. They are, by definition, the standard of care. But, you know, what things physicians have been doing and finding to be useful are the suggestions it's making. It has no expertise. It doesn't read journal articles and doesn't deal with whether or not people believe there were well-done studies or not, it just looks at the EHRs.
Are there any practical complications for the existence of this system?
The only way this is going to work is if all the electronic health records that are relevant to a population of patients are centrally maintained. This only works if you have all the information about a patient, including the final diagnosis. So now, if we have all the electronic health records centralized in place, yes, that's a pretty privacy consideration. The computations required for Bayesian efficient diagnosis are the calculations of those confusion matrices. And that would all be done centrally. It's not, in fact, a vast amount of computing power compared to what's done now in deep learning neural networks, even if you had millions of healthcare records. Because it's a one-time computation. Similarly, the computing at the physician’s side is also completely negligible, in sort of thing easily done on a smartphone. The central system has a very large health record requirement, that's a Cloud Function, substantial, but not unreasonable computational function to extract the confusion matrices, and then an online function to access the queries coming constantly from each physician seeing each patient because they're going to want this information online, they're going to want in the middle of the physical exam, you instead of having to say, you know, where am I now on the physical exam, it would say, these are the parts of the physical exam that you know, are probably your most likely to want to be doing next. And here are the findings you're most likely to be wanting to enter. And instead of having to type them in, it's constantly thinking like you're thinking, and you're making suggestions, which update it on your thinking. And that's not computationally intensive, other than having a good open communication port, with probably much less bandwidth, and we're using it right now for this interview.
Before ending this podcast, Dr. Loeb recommended these three books for reading:
- Guns, Germs, and Steel by Jared Diamond
- Sapiens: A Brief History of Humankind by Yuval Noah Harari
- Wealth and Poverty of Nations by David Landes
Please go through the podcast to know more about his thoughts and views. Timestamps are available for the interview to easily move to the chapter that you wish to learn.