Table of Contents
- Health Inequities and Telehealth - An Effective public health tool Underutilized
- Expert Insights: How to deal with postpartum depression during a pandemic
- Upcoming Course Update
- Decide Wisely - A Guide To Choosing A Medical Specialty - In Post-pandemic Digital Healthcare Era
- The Billionaire Who Controls Your Medical Records
- Swarm Learning for decentralized and confidential clinical machine learning
- Machine learning for sperm selection
- Effect of Implantable vs Prolonged External Electrocardiographic Monitoring on Atrial Fibrillation Detection in Patients With Ischemic Stroke
- From My Twitter; If you Missed
Status
Published
Video
Video
Ready to Publish
Ready to Publish
Publish Date
Jun 3, 2021
Target
Long Form
Blog Ideas
Design
Drafted
Graphics
Graphics
Type
Idea
Video Recording
Health Inequities and Telehealth - An Effective public health tool Underutilized
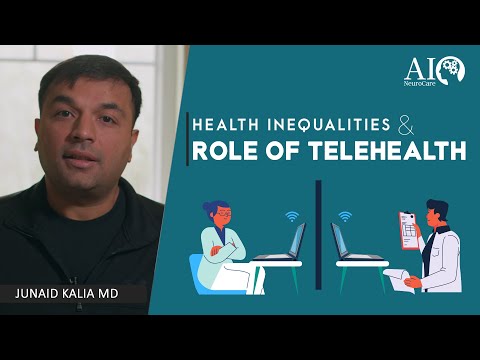
In this video, we will discuss the health inequalities and the role of Telehealth to improve on those equities that are extremely necessary.
Health is a human right but first, we need to understand what health really is. It's a multi-dimensional concept that goes beyond the mere absence of illness. So, the way we determine health is actually a construct that different people use in different ways.
The following are the key promises of Telehealth. 1. Access 2. Allied 3. Analytics
Expert Insights: How to deal with postpartum depression during a pandemic
My Sister @KaliaSaira from University of Arizona discussing:
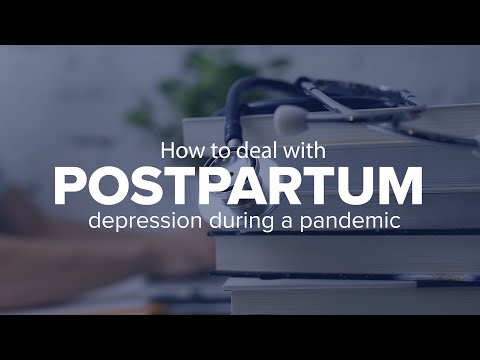
Upcoming Course Update
Decide Wisely - A Guide To Choosing A Medical Specialty - In Post-pandemic Digital Healthcare Era
The pace of change will never be as slow as it is today” - Sue Siegel
Logarithmic to Algorithmic Change - Industry 4.0 to Healthcare 4.0
The Billionaire Who Controls Your Medical Records
“Epic Systems founder Judy Faulkner built an empire pioneering—and later dominating—electronic medical records. For decades, she's kept them walled off from competitors, but now the pandemic is fueling a digital health care race that might finally topple her from the throne”
Swarm Learning for decentralized and confidential clinical machine learning
Fast and reliable detection of patients with severe and heterogeneous illnesses is a major goal of precision medicine12. Patients with leukaemia can be identified using machine learning on the basis of their blood transcriptomes3. However, there is an increasing divide between what is technically possible and what is allowed, because of privacy legislation45. Here, to facilitate the integration of any medical data from any data owner worldwide without violating privacy laws, we introduce Swarm Learning—a decentralized machine-learning approach that unites edge computing, blockchain-based peer-to-peer networking and coordination while maintaining confidentiality without the need for a central coordinator, thereby going beyond federated learning. To illustrate the feasibility of using Swarm Learning to develop disease classifiers using distributed data, we chose four use cases of heterogeneous diseases (COVID-19, tuberculosis, leukaemia and lung pathologies). With more than 16,400 blood transcriptomes derived from 127 clinical studies with non-uniform distributions of cases and controls and substantial study biases, as well as more than 95,000 chest X-ray images, we show that Swarm Learning classifiers outperform those developed at individual sites. In addition, Swarm Learning completely fulfils local confidentiality regulations by design. We believe that this approach will notably accelerate the introduction of precision medicine.
Machine learning for sperm selection
Infertility rates and the number of couples seeking fertility care have increased worldwide over the past few decades. Over 2.5 million cycles of assisted reproductive technologies are being performed globally every year, but the success rate has remained at ~33%. Machine learning, an automated method of data analysis based on patterns and inference, is increasingly being deployed within the health-care sector to improve diagnostics and therapeutics. This technique is already aiding embryo selection in some fertility clinics, and has also been applied in research laboratories to improve sperm analysis and selection. Tremendous opportunities exist for machine learning to advance male fertility treatments. The fundamental challenge of sperm selection — selecting the most promising candidate from 108 gametes — presents a challenge that is uniquely well-suited to the high-throughput capabilities of machine learning algorithms paired with modern data processing capabilities.
Effect of Implantable vs Prolonged External Electrocardiographic Monitoring on Atrial Fibrillation Detection in Patients With Ischemic Stroke
Conclusions and Relevance Among patients with ischemic stroke and no prior evidence of AF, implantable electrocardiographic monitoring for 12 months, compared with prolonged external monitoring for 30 days, resulted in a significantly greater proportion of patients with AF detected over 12 months. Further research is needed to compare clinical outcomes associated with these monitoring strategies and relative cost-effectiveness.