Table of Contents
- Introduction
- Update in Machine Learning in Neurology
- Part 1: Machine learning in Neurology & Healthcare - Trends
- Part 2: Machine learning in Neurology - A technological Based Review
- Disclosures
- Objectives
- Demystifying AI
- AI → ML → DL
- DATA Codes
- Difference Between Statistics and Machine Learning
- Cooking Predictions
- Data Not Model
- DATA > Model
- General Trends in BioMedical AI research
- Automated Machine Learning (AutoML)
- Papers with code - https://paperswithcode.com
- Transfer learning
- Operationalizing Machine Learning for Continuous Learning
- Myth Buster: Continual learning in Medicine
- Why?
- Where is MLOps Used in Healthcare? THE BUSINESS OF HEALTHCARE
- MultiModal Data
- Privacy, Collaboration & Continued Learning
- Federated Learning
- Swarm Learning
- Publications in Neurology related to Artificial Intelligence
- Update in Machine Learning in Neurology
- Part 2: Machine learning in Neurology - A technological Based Review
Status
Published
Video
Video
Ready to Publish
Ready to Publish
Publish Date
Nov 18, 2021
Target
Long Form
Blog Ideas
Design
Drafted
Graphics
Graphics
Type
YouTube
Video Recording
IntroductionUpdate in Machine Learning in NeurologyPart 1: Machine learning in Neurology & Healthcare - TrendsPart 2: Machine learning in Neurology - A technological Based ReviewDisclosuresObjectivesDemystifying AIAI → ML → DLDATA CodesDifference Between Statistics and Machine LearningCooking PredictionsData Not ModelDATA > ModelGeneral Trends in BioMedical AI researchAutomated Machine Learning (AutoML)Papers with code - https://paperswithcode.comTransfer learningOperationalizing Machine Learning for Continuous LearningMyth Buster: Continual learning in Medicine Why?Where is MLOps Used in Healthcare? THE BUSINESS OF HEALTHCAREMultiModal DataPrivacy, Collaboration & Continued LearningFederated LearningSwarm LearningPublications in Neurology related to Artificial IntelligenceUpdate in Machine Learning in NeurologyPart 2: Machine learning in Neurology - A technological Based Review
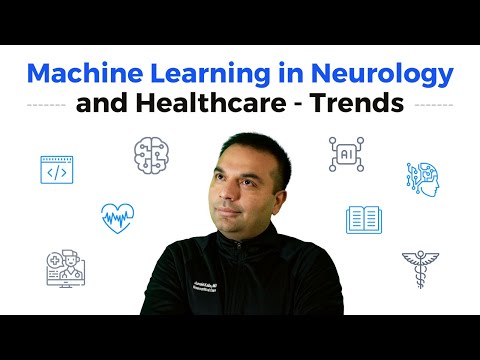
Introduction
Junaid Kalia MD and Adeel Memon will be co-presenting this lecture on Machine learning update in Neurology. Junaid is a neurocritical care, epilepsy and stroke specialist out of Dallas TX. Adeel is movement disorder specialist currently doing his PhD in Neurosciences. Junaid has presented in national and international meetings about role of AI in medicine and Neurology. Here he discusses general trends in Deep learning with specific examples for Neurology and then Adeel will discusses direct specific role of AI in signal processing. Due to time constraints a second portion of the lecture delivery by Junaid will be online only for later review.
Update in Machine Learning in Neurology
Part 1: Machine learning in Neurology & Healthcare - Trends
Part 2: Machine learning in Neurology - A technological Based Review
Disclosures
- VeeOne Health: Vice President Clinical Startegy
- AINeuroCare: Founder
- PINS: Executive Board Member
- APPNA: AI Committee Member
Objectives
The talk is divide into Two Parts:
- Demystifying AI in Healthcare
- Update on AI technologies
Demystifying AI
AI → ML → DL

DATA Codes

Difference Between Statistics and Machine Learning

Machine learning is all about results, it is likely working in a company where your worth is characterized solely by your performance. Whereas, statistical modeling is more about finding relationships between variables and the significance of those relationships, whilst also catering for prediction.
Cooking Predictions
- It is about Predictions
- It is an art as much as science
- It is testing and re-testing till your recipe is Great!

Data Not Model
AI System = DATA + Code [Model]


Tesla is Valued as a Data Company and not a Car company
DATA > Model



General Trends in BioMedical AI research
- Automated Machine Learning (AutoML)
- Transfer Learning
- MLOps
- MultiModal Data
- Privacy, Collaboration & Continued Learning
- Federated Learning
- Swarm Learning
Automated Machine Learning (AutoML)
To make machine learning techniques easier to apply and to reduce the demand for human experts, automated machine learning (AutoML) has emerged as a growing field that seeks to automatically select, compose, and parametrize machine learning models, so as to achieve optimal performance on a given task and/or dataset.




The aim of autoML is to take advantage of complexity in the underlying data set to help guide and identify the most appropriate model (and their associated hyperparameters), optimizing performance, whilst simultaneously attempting to maximize the reliability of resulting predictions

Faes, L., Wagner, S. K., Fu, D. J., Liu, X., Korot, E., Ledsam, J. R., Back, T., Chopra, R., Pontikos, N., Kern, C., Moraes, G., Schmid, M. K., Sim, D., Balaskas, K., Bachmann, L. M., Denniston, A. K., & Keane, P. A. (2019). Automated deep learning design for medical image classification by health-care professionals with no coding experience: a feasibility study. The Lancet Digital Health, 1(5), e232–e242. https://doi.org/10.1016/S2589-7500(19)30108-6

The mean (s.d.) F1 scores across platforms for all model–dataset pairs were as follows:
- Amazon, 93.9 (5.4);
- Apple, 72.0 (13.6);
- Clarifai, 74.2 (7.1);
- Google, 92.0 (5.4);
- MedicMind, 90.7 (9.6);
- Microsoft, 88.6 (5.3).
Korot, E., Guan, Z., Ferraz, D., Wagner, S. K., Zhang, G., Liu, X., Faes, L., Pontikos, N., Finlayson, S. G., Khalid, H., Moraes, G., Balaskas, K., Denniston, A. K., & Keane, P. A. (2021). Code-free deep learning for multi-modality medical image classification. Nature Machine Intelligence, 3(4), 288–298. https://doi.org/10.1038/s42256-021-00305-2



Papers with code - https://paperswithcode.com

Transfer learning
Transfer Learning for Alzheimer’s Disease through Neuroimaging Biomarkers: A Systematic Review
Transfer learning (TL) has turned out to be very popular in the domain of DL, as it enables DL training to be effective in the case of insufficient data

Novel Transfer Learning Approach for Medical Imaging with Limited Labeled Data

Transfer learning is a commonly utilized technique when developing medical imaging models due to a lack of training data. One of the first ideas to use transfer learning was to adopt pretrained models of the ImageNet dataset instead of training from scratch
Transfusion: Understanding Transfer Learning for Medical Imaging
Transfer learning from natural image datasets, particularly ImageNet, using standard large models and corresponding pretrained weights has become a de-facto method for deep learning applications to medical imaging. However, there are fundamental differences in data sizes, features and task specifications between natural image classification and the target medical tasks, and there is little understanding of the effects of transfer. In this paper, we explore properties of transfer learning for medical imaging. A performance evaluation on two large scale medical imaging tasks shows that surprisingly, transfer offers little benefit to performance, and simple, lightweight models can perform comparably to ImageNet architectures. Investigating the learned representations and features, we find that some of the differences from transfer learning are due to the over-parametrization of standard models rather than sophisticated feature reuse. We isolate where useful feature reuse occurs, and outline the implications for more efficient model exploration. We also explore feature independent benefits of transfer arising from weight scalings
Operationalizing Machine Learning for Continuous Learning
Myth Buster: Continual learning in Medicine
Continual learning has long been a part of computer science.1,2 Yet, no medical device based on AI and ML continual learning has yet been approved by the US Food and Drug Administration (FDA)
Vokinger, K. N., Feuerriegel, S., & Kesselheim, A. S. (2021). Continual learning in medical devices: FDA’s action plan and beyond. The Lancet. Digital Health, 3(6), e337–e338. https://doi.org/10.1016/S2589-7500(21)00076-5
- Continuous Integration (CI)
- Continuous Training (CT)
- Continuous Delivery (CD)
Why?
- Data Shift
- Model Shift
e.g. Sepsis Prediction
Effect of a sepsis prediction algorithm on patient mortality, length of stay and readmission: a prospective multicentre clinical outcomes evaluation of real-world patient data from US hospitals

This external validation cohort study suggests that the ESM has poor discrimination and calibration in predicting the onset of sepsis. The widespread adoption of the ESM despite its poor performance raises fundamental concerns about sepsis management on a national level.
The Clinician and Dataset Shift in Artificial Intelligence

Finlayson, S. G., Subbaswamy, A., Singh, K., Bowers, J., Kupke, A., Zittrain, J., Kohane, I. S., & Saria, S. (2021). The Clinician and Dataset Shift in Artificial Intelligence. The New England Journal of Medicine, 385(3), 283–286. https://doi.org/10.1056/NEJMc2104626

Where is MLOps Used in Healthcare? THE BUSINESS OF HEALTHCARE




MultiModal Data
Digital health data are multimodal and high-dimensional

Berisha, V., Krantsevich, C., Hahn, P. R., Hahn, S., Dasarathy, G., Turaga, P., & Liss, J. (2021). Digital medicine and the curse of dimensionality. NPJ Digital Medicine, 4(1), 153. https://doi.org/10.1038/s41746-021-00521-5
Multimodal deep learning models for early detection of Alzheimer’s disease stage
- Imaging (magnetic resonance imaging (MRI))
- Genetic (single nucleotide polymorphisms (SNPs))
- Clinical test data to classify patients into AD, MCI, and controls (CN)


Privacy, Collaboration & Continued Learning

Federated Learning

Swarm Learning

Warnat-Herresthal, S., COVID-19 Aachen Study (COVAS), Schultze, H., Shastry, K. L., Manamohan, S., Mukherjee, S., Garg, V., Sarveswara, R., Händler, K., Pickkers, P., Aziz, N. A., Ktena, S., Tran, F., Bitzer, M., Ossowski, S., Casadei, N., Herr, C., Petersheim, D., Behrends, U., … Deutsche COVID-19 Omics Initiative (DeCOI). (2021). Swarm Learning for decentralized and confidential clinical machine learning. Nature, 1–7. https://doi.org/10.1038/s41586-021-03583-3
Publications in Neurology related to Artificial Intelligence
2018: 77
2019: 70
2020: 172

