Table of Contents
- Home office for the Digital Clinician [2021 Update]
- Investing in Happiness
- How to avoid machine learning pitfalls: a guide for academic researchers
- Simplicity Creates Inequity: Implications for Fairness, Stereotypes, and Interpretability
- Machine Learning in Medical Emergencies: a Systematic Review and Analysis
- Machine learning explained at five difficulty levels
Status
Published
Video
Video
Ready to Publish
Ready to Publish
Publish Date
Sep 2, 2021
Target
Long Form
Blog Ideas
Design
Drafted
Graphics
Graphics
Type
Idea
Video Recording
Home office for the Digital Clinician [2021 Update]
This provides the much needed update to my prior video titled βSetting up an Efficient Telemedicine Workplaceβ. It is astonishing to see one year can bring in terms of consumer tech advancement.
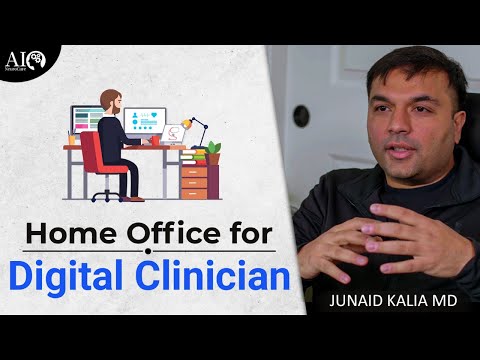
Investing in Happiness
Money has the unique ability to move economic value through time; money made from work done today can be used to fund spending in the future. Time and money are both finite resources that we have to make allocation decisions about on a daily basis
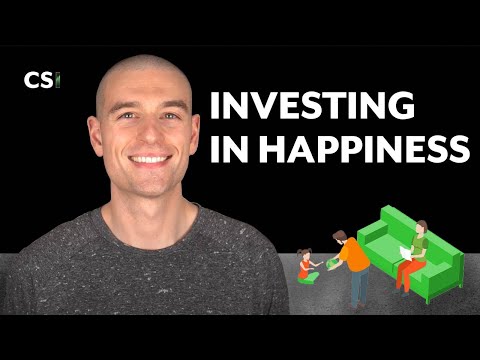
How to avoid machine learning pitfalls: a guide for academic researchers
This document gives a concise outline of some of the common mistakes that occur when using machine learning techniques, and what can be done to avoid them. It is intended primarily as a guide for research students, and focuses on issues that are of particular concern within academic research, such as the need to do rigorous comparisons and reach valid conclusions. It covers five stages of the machine learning process: what to do before model building, how to reliably build models, how to robustly evaluate models, how to compare models fairly, and how to report results
Β
Simplicity Creates Inequity: Implications for Fairness, Stereotypes, and Interpretability
Algorithms are increasingly used to aid, or in some cases supplant, human decision-making .. We develop a formal model to explore the relationship between the demands of simplicity and equity. Although the two concepts appear to be motivated by qualitatively distinct goals, we show a fundamental inconsistency between them.. Thus, simplicity transforms disadvantage into bias against the disadvantaged group. Our results are not only about algorithms but about any process that produces simple models, and as such they connect to the psychology of stereotypes and to an earlier economics literature on statistical discrimination.
Β
Machine Learning in Medical Emergencies: a Systematic Review and Analysis
Total of 20 studies were included in this review. Most of the included studies were of clinical decisions (nβ=β4, 20%) or medical services or emergency services (nβ=β4, 20%). Only 2 were focused on m-health (nβ=β2, 10%). On the other hand, 12 apps were chosen for full testing on different devices. These apps dealt with pre-hospital medical care (nβ=β3, 25%) or clinical decision support (nβ=β3, 25%). In total, half of these apps are based on machine learning based on natural language processing. Machine learning is increasingly applicable to healthcare and offers solutions to improve the efficiency and quality of healthcare. With the emergence of mobile health devices and applications that can use data and assess a patient's real-time health, machine learning is a growing trend in the healthcare industry.
Β
Machine learning explained at five difficulty levels
For their 5 Levels series, Wired brought in Hilary Mason to explain machine learning at five levels of difficulty. Masonβs explanations are super helpful at every level.
Β
Β